Dirty data is among the most significant and costly logistics and supply chain management issues. It contains errors and inconsistencies, which can seriously damage business operations, customer satisfaction, and overall performance.
Dirty data can lead to problems like out-of-stock items and delayed shipments or services, ultimately devastating your client’s business. For instance, the Data Warehousing Institute (TDWI) estimated that, in the US alone, poor, dirty, inaccurate, or missing data costs companies over $600 billion a year.
Accurate and reliable data is crucial for optimizing supply chains in today’s competitive market. This ensures that products or experts are available when customers need them.
In this article, we’ll examine how dirty data affects the supply chain and show effective ways to keep it accurate and up-to-date.
š” For over 15 years, we have created the most comprehensive world postal code database. We update our location data weekly, relying on more than 1,500 sources. Browse GeoPostcodes datasets and download a free sample here.
How Dirty Data Can Sneak Into Supply Chain Operations
Dirty data can often creep into business systems without obvious signs, impacting operations. Various factors contribute to its generation, and some of these might already be lurking in your systems unnoticed:
- Lack of Data Standardisation Across Countries: Operating across borders often means dealing with different formats, conventions, and standards for addresses and zip codes. Data mismatches and errors can quickly settle into your systems without a unified approach.
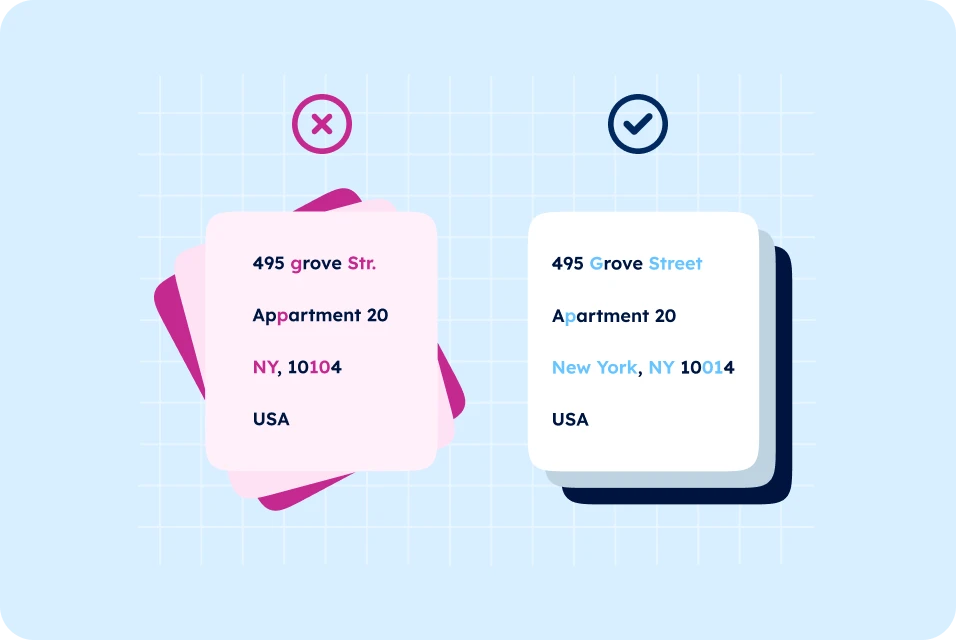
- Hidden Gaps in Data: Datasets may often have missing or incorrect values due to technical or human errors. These gaps will cause issues down the line, such as failed deliveries or inaccurate inventory tracking, which businesses might only notice when they result in operational problems.
- Manual Data Entry Errors: Despite advancements in automation, manual entry is still frequent for many businesses. Human errors, such as misspelled addresses or transposed digits in a postal code, can hinder the quality of your address data.
š” Check out how DB Schenker validates postal data 300X faster and mitigates manual data entry errors.
- False Positives from Multiple Data Providers: A false positive happens when a system says something is right when it’s not. This might mean confirming a wrong address as valid in address validation because the rules set up to catch errors weren’t specific enough. These mix-ups usually happen when filters are too loose or don’t have enough details to catch close-but-wrong matches. The problem with false positives is they clutter up the process with noise, making it harder to trust the location data that should be spot-on.
These factors can create dirty data that silently undermines the efficiency of your supply chain. Identifying and addressing these issues early in the flow can prevent significant operational inefficiencies and optimize your supply chain performance.
How To Overcome Dirty Data Challenges?
Traditional Approaches and Their Limitations
Traditionally, companies might attempt to clean their data internally and implement robust data governance practices in their supply chain design. While these approaches can be effective, they come with significant drawbacks:
- Resource Intensive: Cleaning data and maintaining data governance requires substantial time, effort, and expertise.
- Complexity Across Borders: The challenge is compounded by varying address formats and postal code systems for companies operating in multiple countries.
- Continuous Effort: Data cleaning is not a one-time task. Since zip codes often change, ongoing maintenance is required to ensure data quality, which can be time-consuming and ineffective.
- Scalability Issues: As a company expands into new markets, the complexity of managing diverse data formats increases exponentially. Some countries have very strict location data regulations, such as the case of China, which makes the globalization process even more complex.
Given these challenges, many companies find that internal data cleaning efforts, while valuable, may not be the most efficient solution, especially for global operations.
The Optimal Solution: Standardized Global Postal Code Data
Companies can use comprehensive, standardized global postal code databases from reliable providers like GeoPostcodes to address these challenges more effectively.
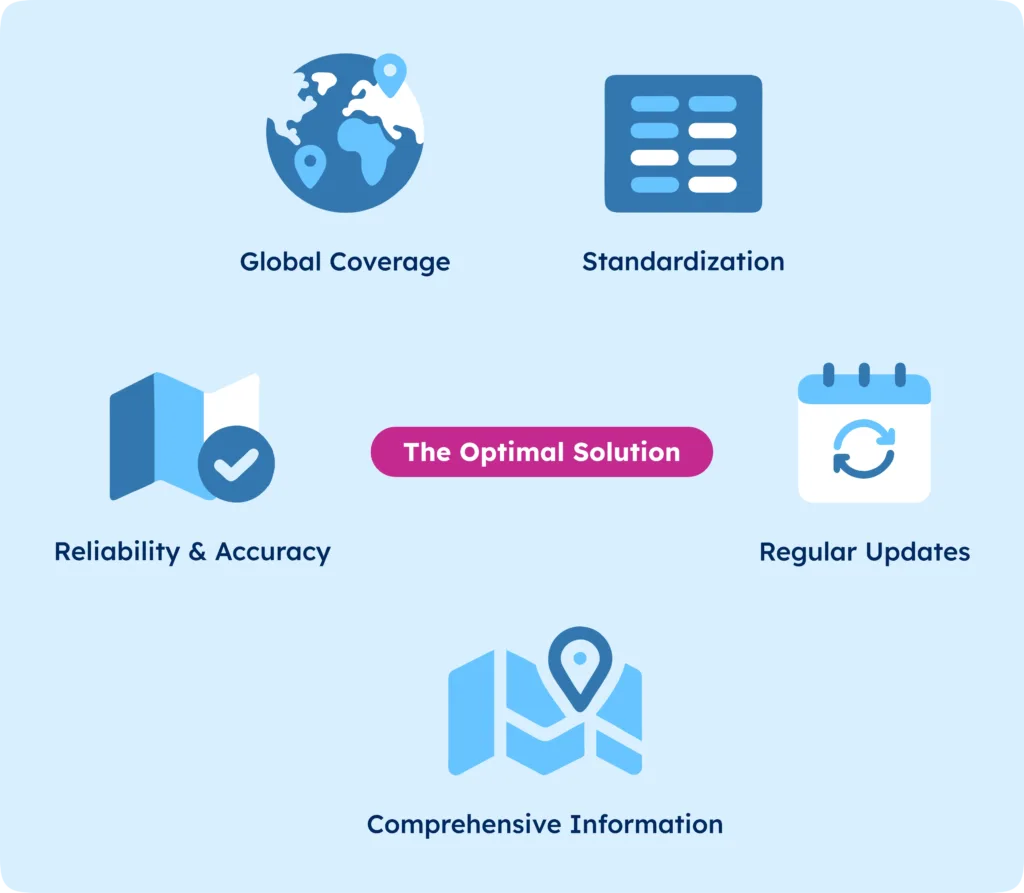
Here’s how this approach helps overcome dirty data:
The ability to effectively harness data is the cornerstone of every successful supply chain. – SupplyChain Dive
- Global Coverage: Opt for a provider that offers zip code data for the entire world, including challenging geographies. This ensures consistency across all your operations, regardless of location.
- Standardization: Use data from a single provider that follows a consistent standardization logic across countries. Different countries and regions have different formats and standards for postal addresses. Address standardization becomes essential to adhere to each country’s local postal standards and improve data quality.
- Reliability and Accuracy: Choose a provider known for reliable and accurate data across different geographies. This minimizes the risk of false positives and hidden gaps in the data.
- Regular Updates: Ensure the data is updated frequently (e.g., monthly) to maintain accuracy and relevance without requiring internal resources for constant maintenance.
- Comprehensive Information: Look for solutions that offer more than just postal codes. For instance, some providers provide postal boundaries, which can be crucial for accurate logistics network design and supply chain digital twins.
Other providers that offer quality location data include TomTom, ESRI, Carto, or Safegraph.
Implementation and Results
By implementing a solution with these characteristics, logistics companies can:
- Compute accurate distances between customer postal codes and the closest warehouses
- Improve delivery-time calculations
- Optimize stock levels in warehouses
- Enhance overall customer service and response times
- Estimate team availability and perform fleet management
- Deliver reliable service for customers who need dedicated support to ensure business continuity.
š” Discover how this multinational technology company optimized Supply Chain performance with reliable Postal code data.
Impact of Dirty Data on Logistics
Increased Operational Costs
Dirty data in logistics can significantly increase operational costs. For instance, errors in order or inventory data can result in excess stock or urgent restocking, which incur additional costs.
Depending on the warehouse size, a company with proper stock levels can spend around 5% of the product cost on the inventory. This cost can be about three times higher if inventory-taking inaccuracies exist. – APQC’s Open Standards Benchmarking database
Moreover, the inefficiencies caused by dirty data can lead to increased labor costs. Employees may spend extra time correcting errors and validating address data, increasing labor costs and diverting valuable time away from revenue-generating activities.
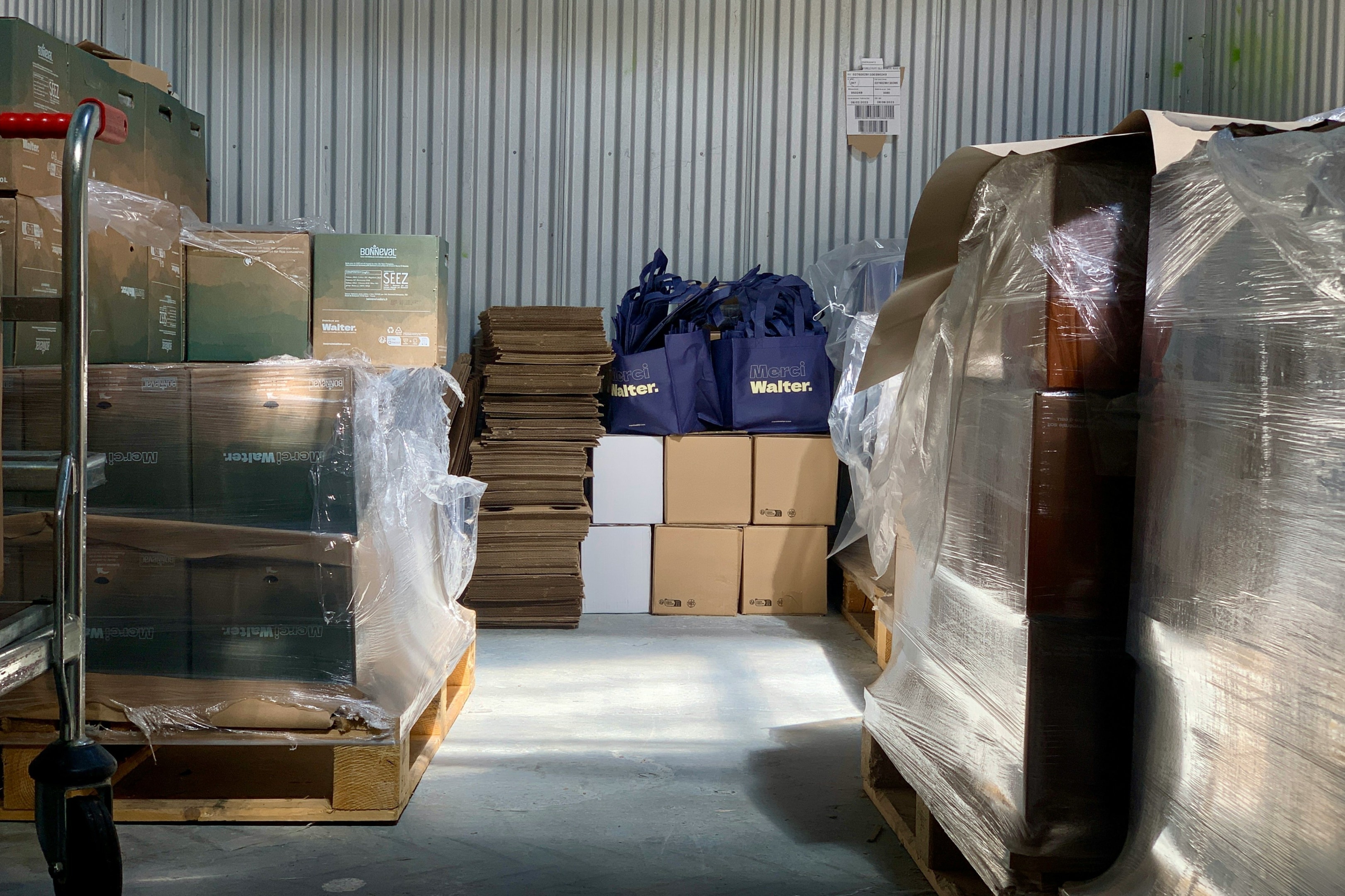
Customer Service Challenges
Dirty data can severely impact customer service, leading to delays, incorrect product shipments, and failed deliveries due to inaccurate delivery-time calculations.
When orders are delayed or incorrect, they reflect poorly on the company’s ability to meet customer expectations, which can have long-term consequences for your customer’s business activity and their trust in your services.
In addition, poor data quality can lead to miscommunication with customers. For example, sending targeted ads within the wrong address radius or contacting customers with incorrect information can further damage trust and confidence in the brand. Ensuring accurate and reliable data is essential for maintaining high customer service standards and building strong customer relationships.
Decision-Making Setbacks
Dirty data can significantly hinder decision-making processes within supply chains. Accurate forecasting, critical for maintaining the right inventory levels and planning deliveries, relies heavily on up-to-date location data.
However, dirty data can lead to inaccurate forecasts, resulting in wrong delivery-time estimations or mismatches between stock and technician deployment.
Furthermore, strategic decisions based on flawed data can be costly to reverse. For instance, if supply chain managers rely on dirty data to allocate resources, they may end up overstaffing or understaffing, leading to inefficiencies and bottlenecks in the supply chain. This affects operational efficiency and impacts the company’s ability to adapt to changing market conditions and customer demands.
Conclusion
Dirty data in logistics leads to significant operational costs, customer service challenges, and decision-making setbacks. Inaccurate data can result in excess inventory, increased labor costs, and missed sales opportunities. It also hinders effective forecasting and strategic planning, impacting a company’s ability to adapt to market changes.
While valuable, traditional approaches to data cleaning often fall short for global operations due to their resource-intensive nature and scalability issues. The complexity of managing diverse data formats across multiple countries makes internal data-cleaning efforts increasingly challenging as companies expand. Continuous maintenance required for data quality further compounds these difficulties.
You can work with a provider like GeoPostcodes to handle those details for you. We maintain a worldwide list of zip codes and related data, from coordinates to administrative divisions, including geocodes and time zones.
We update our data on a weekly basis and employ advanced processing pipelines to deal with the particularities of each country. We enjoy solving these problems, and we’d be happy to save you the trouble!
Reach out to us for further information, or you can directly explore our data from our website.
FAQ
What does a dirty database mean?
Dirty data refers to any data that is inaccurate, incomplete, inconsistent, or outdated, compromising its usefulness and reliability.
What are the key considerations for data cleansing in logistics?
Data cleansing is critical in logistics as it helps eliminate human error and ensures clean data.
Key considerations include identifying and correcting errors, handling duplicates, and updating current data to avoid inaccuracies.
How can artificial intelligence improve data accuracy?
Artificial intelligence (AI) and machine learning algorithms are increasingly used to automate data cleansing processes.
This reduces the time-consuming manual efforts involved in data management.
What are the legal consequences of maintaining dirty data in logistics?
Maintaining dirty data in logistics can have severe legal consequences, especially regarding compliance and regulatory requirements.
Incorrect addresses or delivery information could lead to failed shipments, resulting in potential legal claims.
Moreover, bad data can lead to increased costs and loss of customer loyalty, emphasizing the importance of continuous data cleansing efforts.